Share Via
Real-time data matters if you want to join the AI networking game
Successful solutions based on AI and machine learning rely on the collection of clean and relevant data. Although this seems straightforward, without the data needed to learn, execute tasks automatically, and initiate accurate actions with closed loop automation, these solutions simply will not function as intended. Within this simple idea lies the key to unlocking the full potential of autonomous networking systems: “data matters”.
To help grasp the importance of data, let’s talk about AI for IT operations or AIOps. Early solutions were designed to help organizations primarily solve wireless RF and connectivity issues. Vendors initially collected data that pertained to their hardware platforms to diagnose the RF and performance deviations. The idea of capturing user and endpoint device telemetry allowed for AIOps solutions to expand into more granular troubleshooting and security use cases. But there is a problem with utilizing AIOps to simply summarize reports and dashboards: IT organizations are still left to manually tackle day 2 related issues, although with a summarized task list.
A shift was in the works
While AIOps was helping, these solutions did not capture the entire spectrum of data necessary for comprehensive analysis and use cases that extend from Day -1 to Day N. This led to Gartner expanding on the idea of how utilizing AI in enterprise networking can further help. They outlined new requirements and a shift from using AIOps to AI networking principles.
This new approach enables us to move beyond AI generated report summaries towards closed loop automation with AI. In this model, with the right data, you can create a digital twin of an enterprise infrastructure at the campus and branch, predict deviations in service quality before real issues arrive, and automate improvements towards baseline performance.
While AIOps is able to resolve straightforward and basic issues, it can’t, for example, autonomously address complex network issues, or predict and mitigate sophisticated cyber threats. The problem is that the concept of self-maintenance, optimization, and automation, though not new, has never been integrally implemented at the network layer due to a lack of an integrated data model. Limitations like these leave potential efficiencies and insights on the table and burden IT teams with tasks that, with the right data, could be fully automated.
The promise of an integrated data model
Assuming AI networking could deliver on the idea of day -1 to Day N use cases, IT teams could embrace an operational model that prioritizes visibility and outcomes, thus eliminating the need to invest time and effort in routine operations.
With the resolution of issues that previously demanded extensive investment in time and troubleshooting expertise, IT organizations would be freed to innovate and get to more meaningful projects faster. In fact, the network would become nearly invisible.
Utilizing a digital twin to capture the “essence of the network infrastructure” in real-time is not a new idea but one that has not been truly integrated within the network infrastructure. Digital twin and closed loop automation capabilities that follow simply have not been possible since all enterprise networks have so far been designed with a product mindset: each product with many configuration options, software releases, product models, licensing requirements and complex SKU structure that compromise on performance and security. In the legacy model, the same set of products can be deployed in widely different ways at the hands of different IT professionals, leading to a considerable number of snowflake deployments – hence making it impossible to enable closed loop automation.
Hence, next to an integrated data model, it is important – similar to hypercloud infrastructures – that the campus and branch network infrastructure needs a deterministic system design and standardized architecture that’s purpose built to automatically observe itself and maintain its integrity. Result needs to be an enterprise network that optimizes itself automatically by anticipating and resolving performance issues without manual intervention.
Yes, it’s all about the data
To get that inside-out view of the service, a three-pronged approach to data collection can be taken:
- 360-degree monitoring of all the environmental, user, device, and app context
- Deep instrumentation for every hardware and software component within the network
- Real-time processing with streaming / batch analytics and data-/model-centric AI
Here are a few examples of real-world use cases when it comes to data-first AI strategy:
- Pre-emptively resolved quality deviations in network and user device applications, reducing the need for client action and vendor support tickets.
- Automated software updates and maintenance across the enterprise wired and wireless network, to eliminate operational burden for large-scale network management.
- Significant reduction of risk due to potential malware proliferation and reduction of cyber insurance premiums, by natively embedding zero trust principles within the network.
- Move away from a product-centric break-fix model to a proactive system care, which automates hardware moves, adds and changes towards performance guarantee goals.
- Using device fingerprinting, recognize and protect essential devices within the network to ensure critical applications – like medical equipment – remain uninterrupted.
The Nile difference
It bears repeating: AI is powerless without data. Cloud networking solutions for the enterprise neglecting to integrate AI Networking powered by an integrated data model are at a considerable disadvantage.
At the foundation of the Nile Access Service is the Nile Service Blocks delivering a deterministic system design with campus zero trust security and enabling comprehensive data collection. An as-a-service software delivery model in the Nile Services Cloud ensures that an integrated data model automates traditionally manual day -1 to day N operations across the enterprise network.
And, as the third layer of this unique architecture, Nile’s AI application suite provides the necessary tools to IT admins, their end users, Nile’s service delivery partners, customer success and production engineering teams their own personalized view for full control and visibility.
To catch the Nile Access Service in action to eliminate traditional network operations with AI, check out our closed loop automation demo series.
Share Via
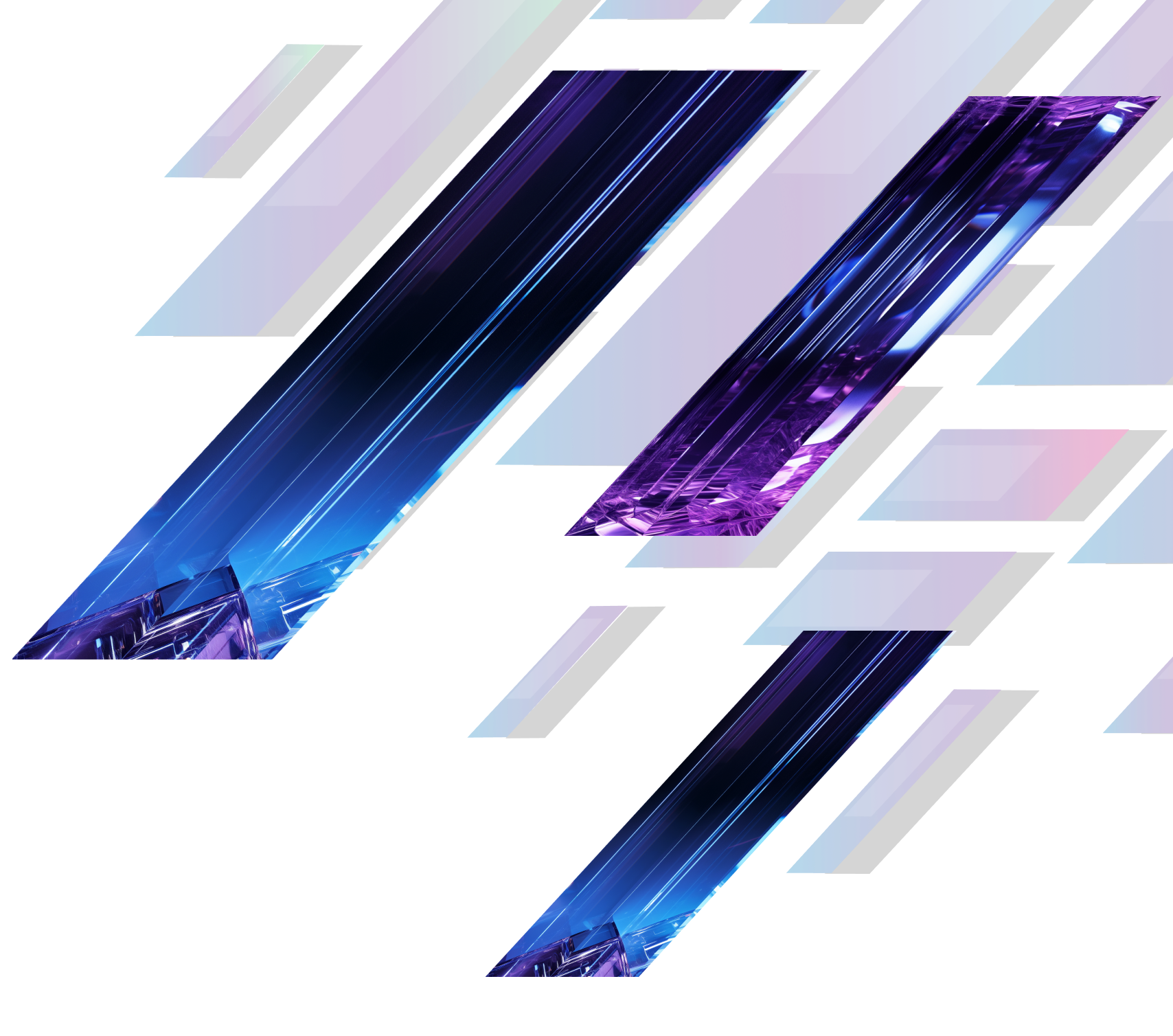