Share Via
Introduction
No other topic today is dominating the discourse in the IT industry like AI. Even after living through the transition to the cloud, the speed with which this technology has become relevant to all aspects of IT infrastructure has been nothing less than breathtaking. As this publication has noted, IT leaders are deeply interested in how AI can make their lives easier.
In this article, I offer a few problem statements that AI can tackle in the enterprise networking space. If you like what you read in this recipe, I would love to hear from you to share more about the ingredients.
Despite the massive hype, AI has room to grow and discover practical applications to tackle. Instead of being a tool for point problems and temporary solutions, industry innovation needs to start moving towards solutions that act like a service that can continue to improve themselves over time. A service that can learn how to tackle problem statements with closed-loop automation. So many solutions fail to deliver on this promise that I have to warn IT leaders out there to be wary of the marketing hype.
If AI keeps telling its users what to do next instead of doing the job itself, could we call that AI?
AI and Enterprise Networks
Within the enterprise networking space, there are several areas for innovation related to AI capable of tackling multiple IT challenges. Most enterprises already need help managing their existing tools; adding more will only exacerbate tool sprawl and spread confusion. It is also important to remember that AI represents a probabilistic model: its accurate depiction of an IT system requires the collection of large volumes of well-designed and highly contextual data to avoid misconfigurations and system outages – causing more pain for an already overwhelmed IT staff.
After seeing many enterprise networks come to life and discussing AI’s impact with IT leaders everywhere, I have concluded that a complete architectural shift is needed to truly leverage AI’s potential within enterprise network infrastructure.
To use an automobile analogy, to design a fully electric vehicle with autopilot software, we can’t expect to retrofit a 1992 Toyota Corolla to make it work. You must form a new design and build with new architecture principles that can translate every bit of the data collected via its sensors to watch the road and take action in software.
With this analogy in mind, let’s take a closer look at today’s enterprise network engineering, security, and operations and then shift to where I think a new model, coupled with AI technology, can successfully deliver an always-on, fully automated, and secure enterprise network.
Today, most AI solutions for the enterprise network across campus and branch locations can be characterized as “AIOps.” This encompasses a cloud management system collecting data from multiple sites, sifting through a wide variety of monitoring and telemetry data, and making recommendations for IT admins to take action. This includes suggestions to IT admins on how to tackle connectivity, device, cabling, and performance issues. While this is undoubtedly useful, for the most part, these solutions simply point out that something is wrong but leave the heavy lifting to the IT staff after reviewing their daily recommendations.
In other words, since today’s networks at the campus and branch are “snowflakes” – each with their own configuration, software releases, and hardware models, as well as many other variables – closed loop automation is simply out of reach with today’s AIOps.
Without a standardized system design for the underlying network, it is impossible for AI to be useful in the last mile and automate the resolution.
Closed Loop Automation with AI
In my opinion, we should demand more from AI to automate operations from Day 0 and onwards throughout the entire network lifecycle. Automation powered by AI will not only point out our problems but also proactively solve them at every stage of the implementation.
As the network is being designed, AI should be able to automatically identify appropriate locations for installing the hardware infrastructure, given the existing network design and outstanding performance requirements. It should automatically maintain a digital copy of an optimized network topology, validating against the installation that’s taking place in real life. Creating a bill of materials and solving the question “what to order” should no longer require hours of manually sifting through spreadsheets and product catalogs – it should automatically be generated by AI.
Once the network is up, interoperability between devices and infrastructure should be optimized without intervention by IT. The network itself should maintain its best practices state, automatically delivering guaranteed performance, coverage, capability, and availability. In the year 2024, especially with the power of AI and closed-loop automation at our disposal, we have to demand guaranteed outcomes from the network.
On an ongoing basis, IT teams should expect the network to continuously tune its own performance, including orchestrating software upgrades, tackling trouble tickets in case of proactive detection of service level deviations, and automating requests for additional capacity and hardware refresh.
In this new world, the traditional Network Operations Center (NOC) for enterprise network infrastructures will no longer exist. For IT teams, monitoring countless product dashboards and alerts would be a thing of the past. Not having to spend countless hours manually managing the network lifecycle would be their new reality.
New Approach, New Architecture
So, how do we get from here to there? From my perspective, we need a few new ingredients to enable IT to fully take advantage of today’s AI capabilities. This new architecture boils down to three core elements:
- A fully integrated network infrastructure delivered as a service, with deterministic and standardized design, eliminating “snowflake” installs.
- A vertically integrated data model that enables real-time observability of the service levels within the network and drives continuous optimization.
- A 360-degree view of the network via sensory infrastructure, powering AI apps that provide IT teams the control and visibility they require.
The idea behind a standardized network architecture is to eliminate the need to build separate configurations with a high degree of variability for every deployment. Short of designing physical infrastructure to determine optimal coverage and performance, each access network should leverage the same APs and switching infrastructure powered by cloud native software.
This is highly differentiated from today’s network model that requires IT to choose from many models of Wi-Fi access points and wired switches, on a building-by-building basis.
Second, establishing a single data model across the entire technology stack is critical to any AI implementation. Unfortunately, many solutions use hardware not built for such data collection and try their best to leverage disparate data formats from older systems. This translates to the primary fear of data scientists everywhere: “garbage in, garbage out (GIGO).” When applied to the data from the enterprise network, the result is poor – or at least unpredictable – network performance that requires manual and reactive troubleshooting.
Finally, 24×7 performance monitoring should be driven by 360-degree monitoring based on deep instrumentation and sensors that monitor both the environment (RF, power fluctuations, cable issues) and the context (users, devices, and apps). These sensors should be embedded deep within the network design, not require manual upkeep, and should be an essential part of any hardware and software component across the system. Data generated by this sensory infrastructure can then be analyzed in real-time by AI applications that can fine-tune the network towards guaranteed outcomes and inform the IT admins and their end users regarding the overall network experience.
Conclusion
There is no reason to expect anything less than closed-loop automation from AI-powered IT infrastructures. To be fair, this transition will take time for many enterprises, the same way businesses took time to acclimate themselves to running their mission-critical applications in the cloud. However, the move to implementing AI to manage the enterprise network is now almost a certainty, much like the shift to the cloud before.
Share Via
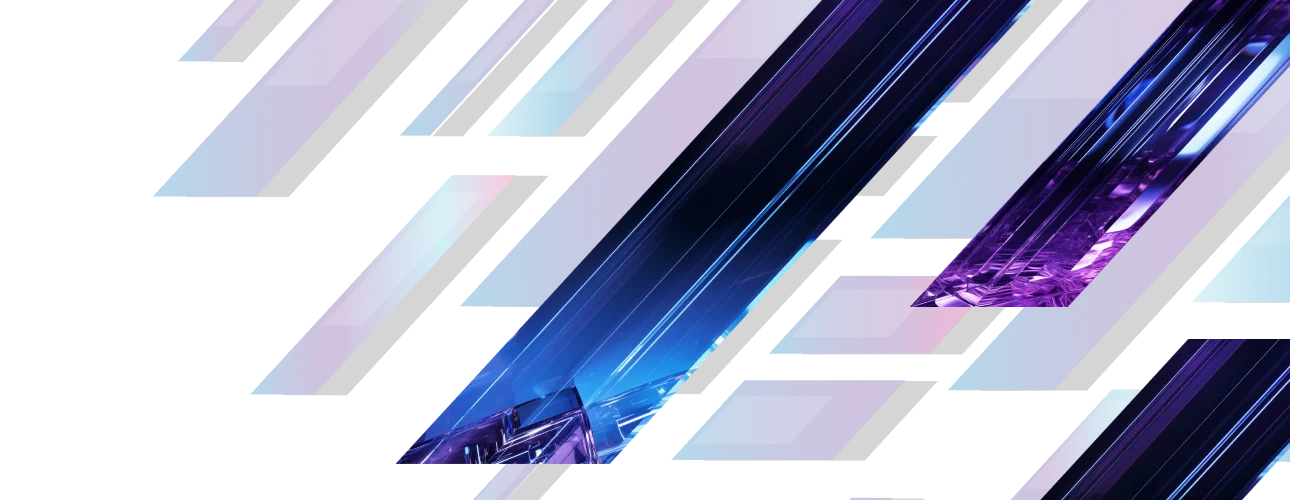